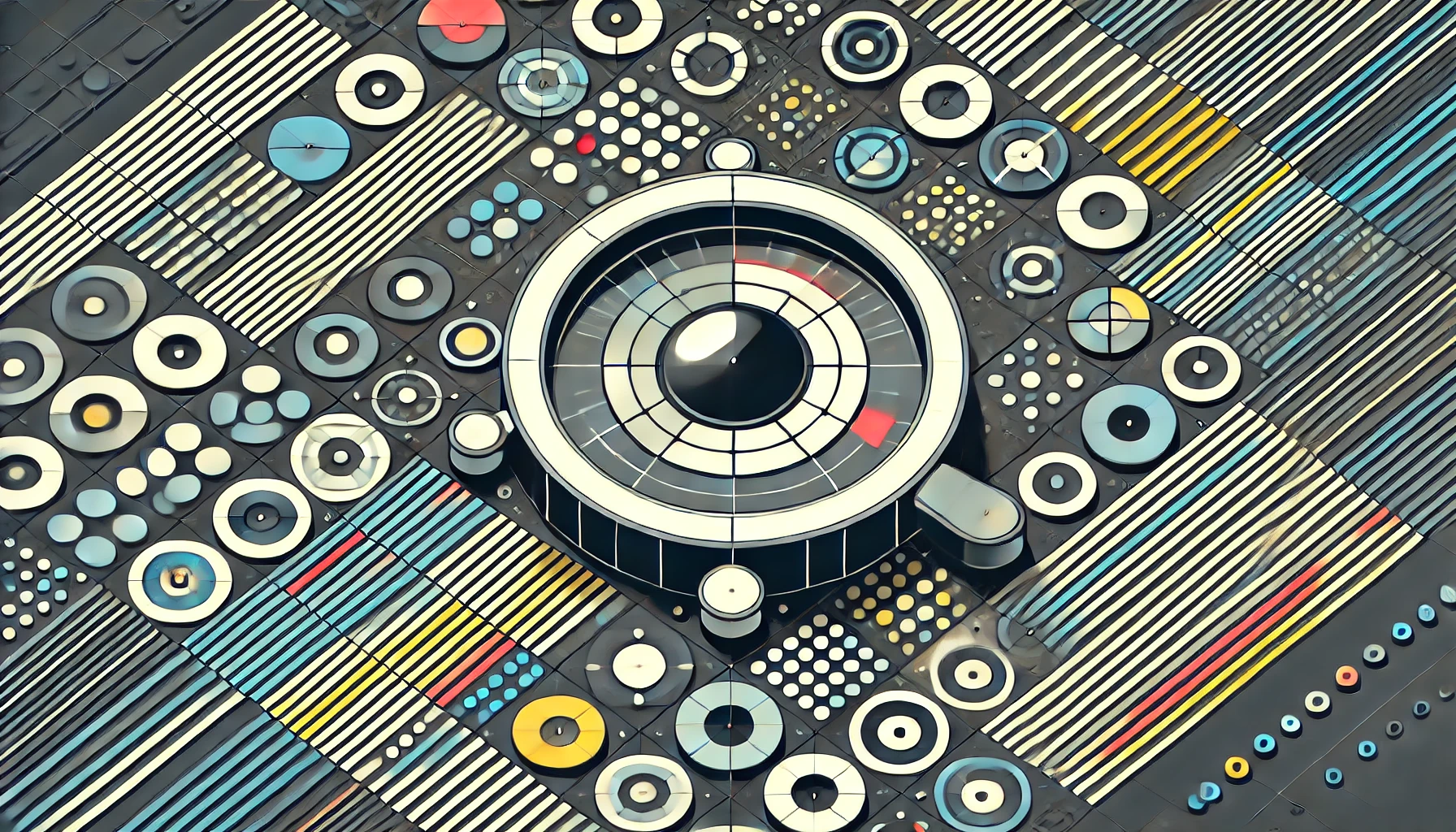
ChatGPT’s attempt to render an Anti-Pattern Detector
Note: In June 2016, I wrote the essay below to capture my thoughts on then-conventional approaches to AI music creation. I didn’t publish it, though some related ideas went into a DARPA proposal that was funded (with colleague Kelland Thomas). Back then, I saw problems with the leading approaches to artificial creativity, viewing them as attempts to conform (to match patterns) rather than to innovate (to break patterns). Rereading this now, in November 2024, with recent attention on Large Language Models (LLMs), Stable Diffusion, and other methods, I still find these ideas relevant—maybe even new (though politically fraught). Rather than leave this on my hard drive, I’m posting it here. Perhaps I’ll revisit it more formally soon.
Multi-Scale Anti-Pattern Detection, or How to Write Tomorrow’s Jazz Today
Ben Grosser
June 27, 2016
History’s efforts to understand music through analysis have led to the development of music theory (and musicology), which offers structured descriptions of how specific composers and musicians have created their works.
Such theories, through history, have not just served as tools of analysis but also as tools for future creation. Music students are taught a system of harmony and chord progression largely based on the past analyses of Bach’s compositions. Advanced courses in music theory examine post-Bach composers (for example, Beethoven, Brahms, Wagner, Mahler, etc.) in order to extend that system. Further extensions are discussed, often as “new” systems, through examinations of 20th-century composers such as Schönberg, Webern, etc.
Computational efforts over the last 20 years have sought to apply these types of analyses to composition and performance databases in order to derive enough fundamental structure from previous works so that it can be used to facilitate the creation of new music as “artificial creativity.” Theorists like David Cope have used such methods to create software that generates “new” Bach, for example.
But these approaches to artificial creativity are missing a fundamental aspect of human creativity: that what makes something interesting and novel is how it differs from what came before it, how it extends the current “system,” and where it defies previously accepted practice.
Moments of difference, extension, or defiance can be seen in all major fields of human knowledge. In science, we think of these moments as paradigm shifts, such as Darwin’s theory of evolution. In literature, we look for moments when the rules change, such as Joyce’s departure from conventional narrative structure in Ulysses. In art, we often talk about these shifts as movements, such as the shift from representation to abstraction, or the turn towards conceptualism.
Such moments are highly communicative, serving to create new conversations that could not have happened previously. Unquestionably, communication happens within a movement, system, or a certain way of thinking. In other words, communication doesn’t require difference, extension, or defiance—but high-density communication (low-context in Hall’s cultural factors) thrives on it.
The music of Miles Davis serves as an illustrative example. Miles got his start playing bebop with Charlie Parker in New York City in the late 1940s. But within a few years, he changed the rules and helped to create the “cool jazz” movement, documented in his album Birth of the Cool. By the late 1950s, Miles changed the rules again, releasing Kind of Blue and another new movement of “modal” jazz. By the late 1960s, Miles extended his work to create “fusion” jazz with Bitches Brew. Over the next six years, his work became increasingly defiant and made many jazz fans angry with albums like On the Corner. In other words, over the course of his career, Miles Davis continually changed the “rules” of jazz, creating new and different styles, extending those styles towards eventual shifts, and often in defiance of established standards and norms (even those he created).
A typical contemporary computational approach to understanding and future creation of music by Miles Davis would be to utilize deep learning to find patterns and structures in Miles’ music. Such pattern detection would then be used to create “new” Miles Davis, usually judged by how closely it sounds like something Miles already played. Such an approach misses the point. To truly create in the style of Miles Davis is to create in a new style, to extend what came before, to defy normal convention. In other words, deriving rules from previous compositions and recordings—and using those rules to generate new compositions—at best gives an average of what came before. This is not innovation; this is imitation.
Instead, what computational researchers need to focus on is what happened between an established style or genre and a subsequent major shift away from it. Instead of looking for patterns in what Miles wrote and played, we need to find the anti-patterns—the moments of extension when what came after was distant from what came before, the shifts of thinking that others saw as breaking the rules (and often, as a loss of direction).
In other words, instead of a pattern detector, we need an anti-pattern detector—a way of finding the innovative transformations that led to major shifts in the history of arts, science, and engineering. With such a system, we could not only produce new work that is not simply an average of the old but could theoretically produce the next album Miles Davis would have written—a new shift that many would see as a wrong turn (until they eventually see that it’s right). Such a system could produce the jazz, literature, or science of the future.
These moments I’m describing are some of the most communicative in history. They led to new ways of thinking, hearing, seeing. While plenty of useful communication happens within current systems of thinking, within existing patterns of interaction, within the “rules,” it is those moments outside of the rules that say the most with the least. These moments help us to see the systems we were living within but could not see at the time. They help us to understand why we do what we do. We can use these moments as subjects of anti-pattern analysis to derive new methods of transforming history and the present into the ideas of the future.